AI Safety and Action: How Governance Builds Trust
The current AI wave holds remarkable opportunities for innovation, societal advancement and economic growth. To realise this promise, companies are securing massive amounts of compute, energy, data and other resources necessary for AI advancement. But the commercial and societal success of AI hinges largely on another resource not often discussed – trust.
Trust encompasses multiple dimensions, including public confidence, system reliability and transparency. But how can we cultivate this critical trust? The answer may lie in effective and targeted governance. If AI is not secure, reliable, and transparent, the risk of harm increases, users lose trust in AI tools and adoption of the technology may stall.
By looking at key themes such as transparency and bias, and examining their evolution in other sectors, we can better understand the risks that need to be mitigated and, importantly, the benefits – to both safety and innovation – of implementing an effective governance program.
This article (PDF Version) is authored by Brendan Kelleher, Partner and Chief Compliance Officer at SoftBank Group International and Nicole Kidney, Senior Associate at Clifford Chance.
Trust in AI: Insights from the Paris AI Action Summit
The Paris AI Action Summit was the third meeting of heads of state and tech leaders on the topic of AI. While earlier events – Bletchley Park, UK in 2023 and Seoul in 2024 – focused on AI safety, the Paris Summit broadened the conversation by emphasising AI's role in driving innovation. Despite this shift, a central theme persisted in Paris – "trust in AI" is the foundation to both safety and progress.
Throughout the AI Action Summit and fringe events, participants stressed the importance of building societal confidence in AI as a means of accelerating innovation. Many proposed achieving this through a common goal – shared among developers, regulators and the public – of establishing AI for public good. Attendees identified the need for public transparency and accountability to improve understanding of, and trust in, AI. Some underscored the benefits of a common language on AI governance and safety to better align the expectations of all stakeholders.
Lessons from Other Sectors
Which brings us back to the crucial question – how can this essential trust be earned? History offers examples where governance has been used to foster trust in sectors initially faced with scepticism and fear. These governance frameworks not only protected the public but also unleashed a wave of innovation, transforming the once-hesitant products into integral parts of daily life.
The pharmaceutical industry has long been held to strict safety protocols. Ethics committees ensure that pharmaceutical products are not released without being assessed by an impartial, diverse group charged with protecting the rights and safety of individuals. Similarly, rigorous clinical testing helps to ensure products come to market safely and post-market surveillance validates product safety once in widespread use. These governance mechanisms have helped to promote industry transparency, accountability and build trust.
The automotive industry's type approval regulations are another successful model for applying technical and safety standards to ensure reliability. Customers are reassured knowing that vehicles have undergone extensive testing to prove their safety, even when new advanced features are introduced.
The aviation industry has also earned trust by embedding transparency and accountability into its governance framework. Incident reporting and black boxes play a big role in this. When an incident occurs, airlines are required to document and investigate it. These reports are shared across the industry and reported publicly, creating a collective learning system and common language. Every airplane is equipped with a black box that records flight data to further help explain to regulators, airlines, manufacturers, and the public what went wrong and how to prevent recurrence.
Each of these industries demonstrates that effective governance does not stifle innovation – it enables it. A similar approach in AI, combining proactive safety measures, transparent reporting and common industry standards could help bridge the trust gap.
Challenges to Building Trust in AI
While the benefits of building trust are well-recognised, the AI sector faces unique challenges. These need to be navigated carefully to ensure that governance is straightforward, effective and continues to foster innovation. Companies and regulators are grappling with these issues today, another common theme from the AI Action Summit.
Transparency and Confidentiality
Transparency is key to earning trust; but it comes with its own obstacles. To improve public confidence in AI, users and regulators increasingly expect developers to provide them with the necessary information to understand AI systems and their outputs. However, the inherent complexities of frontier AI models make them difficult to explain in a user-friendly way.
AI companies also have disincentives to revealing the inner workings of their products. For example, sharing the technical workings behind AI systems could expose vulnerabilities, allowing for malicious attacks, or facilitating reverse engineering.
Bias and Accuracy
The public has heard much about how AI systems can perpetuate human bias by reflecting prejudices inherent in their training data. Instances of AI hallucinations, and the consequences of relying on such inaccuracies, have also been widely reported in the press. Risks of bias and inaccuracies in AI outputs undermine public confidence in AI.
AI companies have made strides to address hallucinations, using strategies such as chain of thought reasoning and consistency checks to, in essence, double-check the work of models. But more public education is needed on the utility and accuracy of AI to counter its reputation for mistakes. This is an area where a common language may emerge.
While hallucinations are a technical issue addressed with technical solutions, bias is an issue systemic to society. Addressing it has proved challenging because bias is inherent in the data on which models are trained. Our concept of bias is also a moving target. Nevertheless, technical and procedural methods to mitigate bias can be deployed at all stages of AI development. Success on this front is essential to advance trust in AI.
User Empowerment
The enigmatic nature of AI can cause scepticism and misconceptions. This scepticism can often hinder adoption of AI products in everyday life. In addition, AI-related incidents can quickly exacerbate these fears and erode trust.
The more users understand why AI acts in a certain way and can rely on AI developers to investigate and remediate incidents, the more they will trust it. Companies should think carefully about how they can educate users and build AI literacy into their communication strategies. Similarly, enterprises can train their employees on AI’s risks and opportunities, helping to develop a shared understanding and common AI language. Publicly prioritising safety through rigorous testing, incident reporting and clear accountability frameworks can further empower individuals to make informed decisions and use AI responsibly.
What's Next?
Building trust is not just a regulatory checkbox exercise but a strategic goal that benefits all stakeholders.
A “trust infrastructure”, established through effective and targeted governance, is critical and helps to address three challenges: first, managing the risk of harm as companies build secure products; second, increasing user adoption through promoting trust, safety and well-being; and third, clarifying developer and user expectations to create space for innovation. Governance then becomes an enabler of sustainable growth, aligning the objectives and strategic goals of all stakeholders in the AI ecosystem.
The result? A future where AI is not only powerful and transformative but also trusted and embraced by society.
Authors
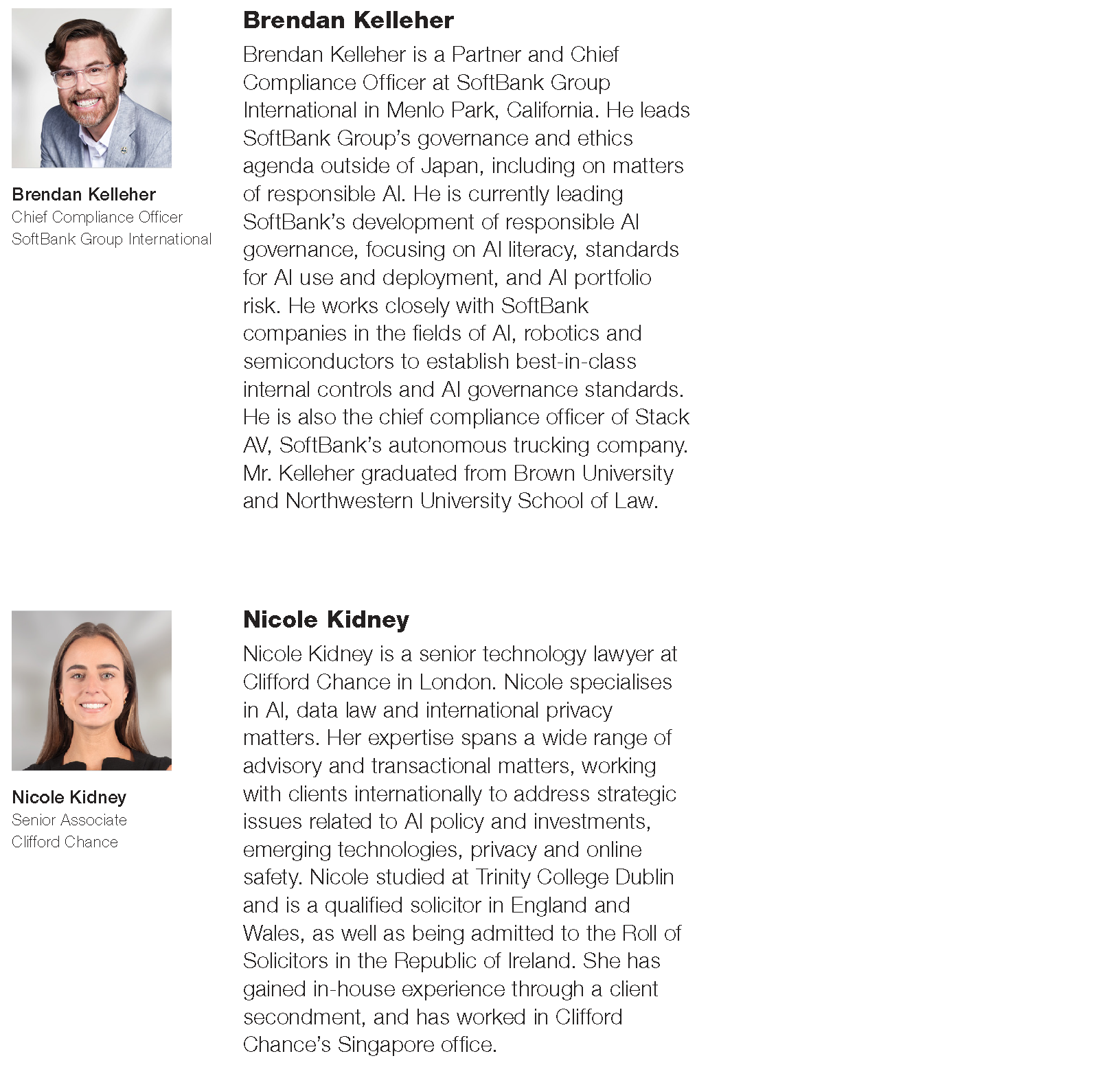